Laasmaa M, Vendelin M, Peterson P
J Microsc 2011 Aug;243(2):124-40
PMID: 21323670
Abstract
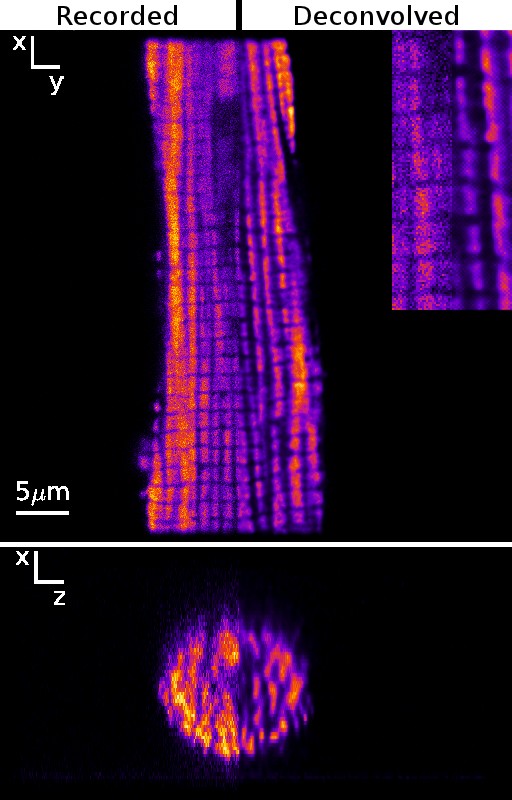
Although confocal microscopes have considerably smaller contribution of out-of-focus light than widefield microscopes, the confocal images can still be enhanced mathematically if the optical and data acquisition effects are accounted for. For that, several deconvolution algorithms have been proposed. As a practical solution, maximum-likelihood algorithms with regularization have been used. However, the choice of regularization parameters is often unknown although it has considerable effect on the result of deconvolution process. The aims of this work were: to find good estimates of deconvolution parameters; and to develop an open source software package that would allow testing different deconvolution algorithms and that would be easy to use in practice. Here, Richardson-Lucy algorithm has been implemented together with the total variation regularization in an open source software package IOCBio Microscope. The influence of total variation regularization on deconvolution process is determined by one parameter. We derived a formula to estimate this regularization parameter automatically from the images as the algorithm progresses. To assess the effectiveness of this algorithm, synthetic images were composed on the basis of confocal images of rat cardiomyocytes. From the analysis of deconvolved results, we have determined under which conditions our estimation of total variation regularization parameter gives good results. The estimated total variation regularization parameter can be monitored during deconvolution process and used as a stopping criterion. An inverse relation between the optimal regularization parameter and the peak signal-to-noise ratio of an image is shown. Finally, we demonstrate the use of the developed software by deconvolving images of rat cardiomyocytes with stained mitochondria and sarcolemma obtained by confocal and widefield microscopes.